Machine Learning
With technology nodes continuing to shrink, wafer structure – with its increasing levels of ambiguities – has become more complicated.
Nova is continuously developing deep machine learning capabilities enabling the company’s tools to learn without human programming. This multidisciplinary area involves computer and mathematic sciences, statistics, data mining, and information theoretic approaches. Nova’s innovative and unique approach to Machine Learning models enables the development of state-of-the-art solutions specifically tailored to the needs of the semiconductor industry.
Nova’s Machine Learning algorithms extract valuable information from input data regardless of training sample availability. In addition, they simultaneously extract accurate information, while maintaining the smallest possible computation load and training set size.
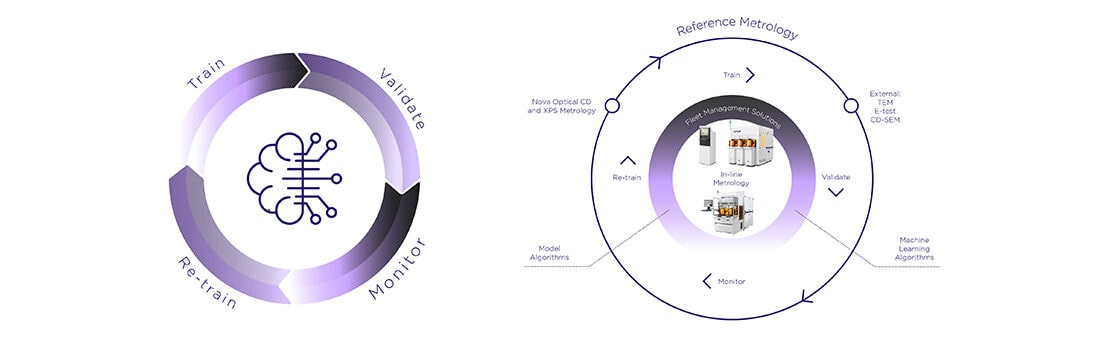

Why Machine Learning?
As technology nodes continue to shrink, wafer structure – with its increasing levels of ambiguity – has become more complicated. Though physical modeling is the measurement method of choice, a model-less approach such as machine learning may provide a more rapid solution.
How it Works?
Machine learning receives input data and corresponding responses, and then learns the relationship between them. The learning process is an optimization problem that minimizes errors between the predicted output and reference response. The output is an estimator used to predict the future response of unseen data. Suggesting a correct tunable mapping of input into output is the root of the process: Nova’s vast experience with model-based approaches helps devise these mappings.
By perusing the most accurate prediction, the algorithm developer needs to determine the type of algorithm (e.g. neural network, support vector machine, PCA) and the optimized set of hyper-parameters. Deep knowledge of Nova’s tools enables the company to characterize expected tool noise characteristics at an unprecedented level of accuracy.
Since training, validation and testing are done at the customer’s site, Nova’s algorithm needs to be fully automated. The hyper-parameter selection algorithm is generic and versatile enough to produce correct predictions on data coming from different technology processes and measurement tools. The solutions are also designed to be robust to variations in training set sizes. These requirements are achieved while maintaining the accuracy demanded by customers.